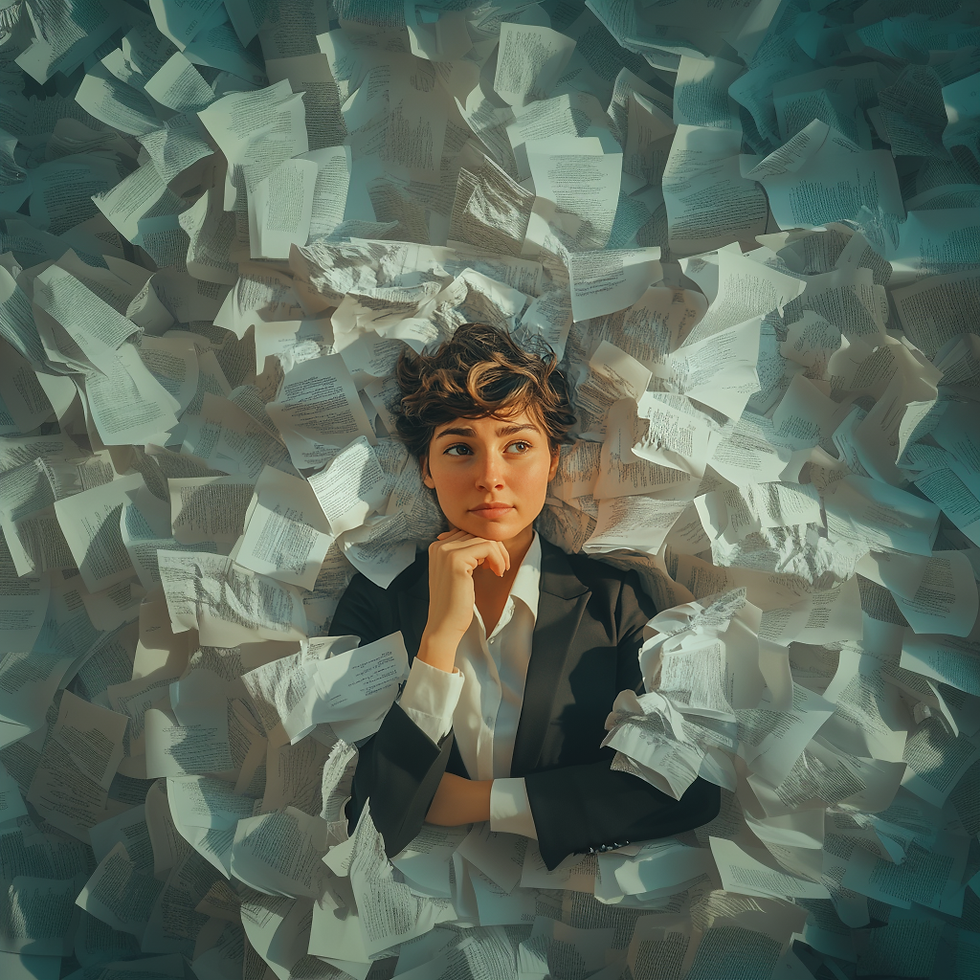
Generative AI (GenAI) is making waves in the enterprise world. Companies are racing to integrate AI Co-pilots into their workflows, eager to enable their employees with the creativity of foundational AI. Co-pilots generally use retrieval augmented generation (RAG), drawing on the vast knowledge stored in the company’s systems to produce highly tailored and contextualised results.
What are RAG models? Retrieval-Augmented Generation is a technique in AI that combines two components: a generative model and a retrieval mechanism. The generative model, like GPT, creates content based on input data, while the retrieval system pulls relevant information from external sources (such as databases or knowledge systems). This allows the AI to generate more accurate and context-aware responses by grounding its outputs in specific, real-time data rather than solely relying on pre-trained knowledge. |
But here’s the catch: while every organisation wants the benefits of GenAI, nobody wants it rummaging through every corner of their data stores, particularly if that data has been gathering dust for years. Firms need a way of determining which, among their millions of files and trillions of data points, are safe for any given GenAI application to access, which to avoid and which to remove altogether.
Hence, firms face a classic case of snog, marry, avoid—better titled clean, avoid, remove— in which firms have important decisions to make in order to ensure their GenAI rollout is a compliant success:
Clean: Ensure that data accessible to GenAI is both safe and relevant to the specific model application. This process involves preparing and validating the data to ensure compliance and appropriateness before allowing the AI to interact with it.
Avoid: Some data might not be suitable for GenAI due to its sensitivity, irrelevance, or regulatory constraints. However, this data may still need to be retained within the organisation's repository. In these cases, ensure that the GenAI systems are configured to exclude these datasets from both training and retrieval processes.
Remove: Large organisations often store outdated or problematic data that could pose risks during AI rollouts. It’s essential to proactively remove such data to avoid future non-compliance and mitigate the chances of inappropriate information being surfaced by the AI – as long as you can do so without falling foul of your retention obligations.
At the scale of the enterprise and with the complexity of data governance, this is easier said than done. Further, once the model is in production, firms need real-time visibility into what data is being accessed and when. Without these steps, the risks of GenAI implementation—compliance, operational and reputational—are severe.
Therefore, to safely leverage GenAI as an enterprise, strong data governance is paramount. We recently spoke with Castlepoint to understand how they help organisations gain control over their data, ensuring that AI only draws from the right data, at the right time.
Control the controllables
Foundational GenAI models are black boxes, and the same is true of RAG models that leverage these underlying infrastructures. Firms can’t fully explain how or why decisions are made, but they can control the inputs (the data) that these models have access to.
Before implementing GenAI copilots, firms need a clear understanding of their data: where it’s stored, its risk profile, how accurate and current it is. Without this transparency, companies are always going to be on the back foot when it comes to managing AI risks including hallucinations, bias, and data breaches.
Reduce hallucination risk
GenAI models are known to hallucinate, producing incorrect or irrelevant information in otherwise convincing outputs with full confidence. The problem is made worse when RAG models pull from outdated or irrelevant data sources, leading to decisions based on flawed inputs.
Auditing every data point in an organisation is a daunting task, but Castlepoint is simplifying this process with automation, helping firms understand exactly what data they have and where the risks lie.
Metadata extraction: To prevent hallucinations, you first need complete visibility into your data. Castlepoint scans unstructured content like emails, chat messages, and documents, extracting key metadata (names, dates, regulatory phrases) from the actual content, as well as the properties. This creates a structured, dynamic registry of your data, granting you full transparency into what’s in your environment.
Input transparency: Castlepoint tracks who is calling Gen AI, and exactly which documents the AI has used to form its response. It shows what terms, topics, and people are in the document, and who has been interacting with it and when. This ensures full visibility into where AI is pulling information from. If a hallucination does occur, you can trace its source to outdated or irrelevant data, and take corrective action.
By organising and auditing data before AI access, firms can significantly reduce the risk of AI hallucinations and ensure that the decisions made by their systems are grounded in clean, relevant data.
Reducing bias: ontologies for fair representation
GenAI can also amplify bias, whether overt (toxic language) or subtle (underrepresentation of certain groups). Tackling bias is challenging, but Castlepoint uses ontologies—formal models of knowledge that define entities, concepts and their relationships—to organise data systematically and flag potentially biased content. Navigating data through ontologies is inherently intuitive, and allows organisations to explore data according to their unique risks and areas of sensitivity.
Ontology-based data management: Castlepoint detects bias by flagging risky phrases or content based on predefined value-based or organisational ontologies. This can identify sensitive attributes like gender, political persuasion, religion, or other demographic factors, or topics of specific concern or interest to the organisation, enabling firms to clean, avoid or remove these data points in the implementation of RAG models.
Quantifying bias in GenAI outputs is extremely difficult, but by providing transparency into where biases may exist within training and reference data, organisations are empowered to take proactive steps to mitigate bias in their AI systems, promoting fairer and more inclusive decision-making.
Manage privacy risks
Privacy concerns add another layer of complexity to GenAI deployment. AI models can inadvertently surface sensitive personal information, especially when working with poorly governed data sets. Accidental exposure of personal or confidential data—even via AI-generated outputs—can lead to costly GDPR (or equivalent) violations and severe reputational damage.
Sensitive information detection: Castlepoint excels at detecting where personal identifiable information (PII) and other risky content is stored within your systems. Once flagged, firms can either exclude this data from their AI’s training pool or carefully monitor AI interactions with PII, ensuring it is not inadvertently surfaced in response
Data minimisation: Castlepoint automatically, transparently, and accurately applies records retention schedules to content, in any system and format. This means that records that no longer need to be retained can be compliantly disposed of, in accordance with privacy and other relevant laws.
Robust data governance as a foundation for successful Generative AI deployment
Generative AI has the power to revolutionise the way enterprises operate, providing unprecedented creativity and efficiency through AI Co-pilots. But this innovation comes with its own set of challenges, especially concerning data governance. Organisations must carefully navigate which data is fit for AI interaction, which must be restricted, and which is best to eliminate entirely.
The stakes are high. Without thoughtful, proactive data governance, companies risk non-compliance, reputational damage, and AI systems making decisions based on flawed or biassed information. To safely leverage the transformative capabilities of GenAI, enterprises need transparency, control, and the right tools.
Castlepoint offers a comprehensive approach to these challenges by automating data auditing, ensuring visibility into data usage, and applying ontologies to manage bias. By providing full transparency into the data that feeds AI models, Castlepoint allows companies to control the inputs to their AI systems, minimising risks like hallucinations, bias, and privacy breaches. This approach transforms the challenge of data governance from a daunting obstacle into a strategic advantage.
As companies consider GenAI rollouts, they must prioritise robust data governance—ensuring AI draws only from relevant, well-governed data sources. This disciplined approach empowers enterprises to unlock the full potential of generative AI, turning their AI initiatives into true drivers of innovation and success.
Get involved
Are you ready to become a thought leader? Reach out to discuss our ongoing research initiatives, how they impact your firm and where we can work together to position you at the forefront of your industry.