In their 2024 research report, Generative AI in Risk and Compliance: Friend or Foe?, Parker & Lawrence examine the impacts and risks of emerging AI capabilities across 14 RegTech use cases, with insights from 17 expert contributors.
Among those use cases is ESG data & ratings:
ESG Data & Ratings Tools
ESG Data & Ratings providers aggregate, and in some cases, score, publicly available and privately sourced data on the environmental, social and governance performance of companies. Tools in this space leverage AI and machine learning combined with extensive human quality control to provide clients with a holistic, dynamic and reliable view of companies, from which they can base a variety of business and investment decisions.
Key Generative AI Use Cases in ESG Data & Ratings
Expert contributors helped map the opportunities, risks and imminence of 400+ Generative AI applications. The mapping of ESG data & ratings opportunities shows 5 impactful Generative AI capabilities:
Labelling Existing Data: The application of generative AI to automatically assign meaningful labels or tags to data, potentially unstructured or semi-structured, enhancing data organisation and usability for machine learning models.
Interpreting & Summarising Information: Utilising Generative AI to digest and condense large volumes of data into concise summaries, providing insights and overviews that aid in decision-making and comprehension.
Comparing Documents: The capacity of Generative AI to analyse and highlight differences or similarities between various documents, aiding in tasks such as version control, plagiarism detection, and legal document analysis.
Creating Reports: The use of Generative AI to create detailed, structured reports based on input data. This includes comprehending the potentially formal and official nature of regulatory reporting.
Client Interactions: The deployment of generative AI in interactive applications such as chatbots or guided workflow tools to simulate human-like conversations, providing support to end-customers.
Of which 2 have already seen Generative AI implemented or will do imminently.
Impact vs Readiness
Want to dive deeper? Check out the full report for expert commentary:
Key Generative AI Risks Mitigated through ESG Data & Ratings
The analysis also accounts for the emerging risks which RegTech solutions will need to solve. The mapping of ESG data & ratings finds that it can contribute towards solving 4 risks:
Hallucinations: The phenomenon of generative AI models producing false or fabricated information that appears credible.
Bias: The inadvertent reinforcement or creation of prejudiced outcomes by Generative AI. This encompasses any form of partiality, discrimination, or unfair weighting in the information or decisions generated by AI.
Malfunctioning Systems: The risk that complex Generative AI systems may behave unpredictably or fail to perform as intended, without detection, due to their opaque nature. This also includes the knock-on effects on surrounding systems.
Compute Power: The significant computational resources required to train and run generative AI models, which can lead to environmental concerns, increase the barrier to entry, and potentially centralise power.
However none of these are imminent concerns.
Severity vs Imminence
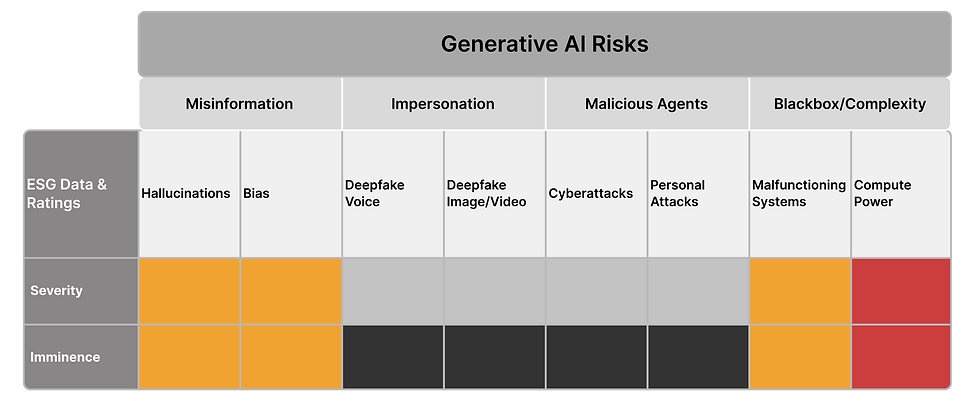
The Industry's Perspective
Throughout the research, experts from technology vendors, regulated institutions and regulators share new insights on Generative AI in risk and compliance. Thank you to OWL ESG for contributing an industry perspective on Generative AI in ESG data & ratings.
To keep this blog bitesize, here is just one excerpt inspired by OWL's insights:
The AI Workflow for ESG Data & Ratings
OWL uses a multi-AI Model approach within the data extraction and aggregation process. Their tools extract snippets from various sources on topics important to users. The data is then extracted from these snippets, providing a trail back to the original sources. The extracted data and information is then used to generate consumable pieces of content, ensuring high quality and accuracy.
What might this look like in practice?
Typically, database queries return standardised answers, presented within the fixed parameters of the query itself, and it is the job of the analyst to contextualise these data points. Generative AI promises to automate much of this contextualisation. The example below illustrates a human-like Generative AI response:
Question: Has the company set targets or objectives for emission reduction?
Answer: Yes, the company has targets and goals for emissions reduction. They have seen positive progress in their waste diversion rate and are driving progress towards their 2030 targets. They are focussed on identifying and testing innovative solutions that can be scaled across their global operations and engaging with their value chain to reduce GHG emissions. Additionally, their GHG reduction goal has been validated as science-based by the SBTi, confirming alignment with a 1.5 degree Celsius pathway.
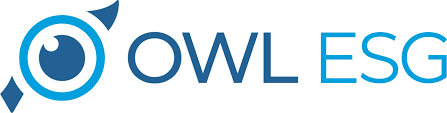
OWL ESG provides clients with the data and tools to make ESG-related decisions with confidence. This includes delivering accurate and objective ESG data for investors and stewardship teams, as well as providing corporates and their consultants with data to evaluate strategic priorities against peers and market trends.
About Parker & Lawrence
At Parker & Lawrence, we are passionate researchers with extensive experience in regulation, risk, and technology. Our unique blend of market analysis and collaboration with regulators, technology vendors and their buyers positions us at the forefront of industry insights. We specialise in AI, RiskTech and RegTech, forming dynamic partnerships with clients to elevate their marketing strategies.
Ready to become the thought leader of your industry? Let’s connect and explore how we can drive success together.